FEATS
A framework to ensure and assess trustworthiness in sensor systems
Framework to Ensure and Assess Trustworthiness in Sensor Systems (FEATS)
The Situation
Current trends suggest that sensors will provide intelligence, surveillance, and reconnaissance (ISR) and decision support “on demand” to warfighters in future operations, without the need for tasking or querying individual sensors. Before this can happen, however, the reliability and trustworthiness of existing and envisioned sensor networks must improve during operations: the sensor network must be robust to deception, node compromise, and various other attacks, while maintaining the operator’s situational awareness regarding the health and integrity of the system.
The Charles River Analytics Solution
We designed, implemented, and evaluated FEATS to analyze sensor-related data and assess the trustworthiness of sensors within a sensor network. FEATS allows a sensor network analyst to track the trustworthiness of sensors without the need for any dedicated diagnostic channels. Instead, data classifiers compare new data to previously observed behavior, to identify anomalous and potentially untrustworthy behavior. The framework enables rapid customization of classifiers for a specific sensor network deployment. To increase overall classification accuracy, multiple classifiers can be combined in parallel or series. This versatile approach to classifier composition also allows a FEATS-based application to compare results between sensors and groups of sensors, which allows the application to scale to very large networks. A trustworthiness assessor aggregates the output of the classifiers to infer the trustworthiness of each sensor or group of sensors over time. Because the trustworthiness of a sensor may need to be inferred from multiple classifiers, the assessor performs a weighted aggregation of classifier results based on the confidence of each classification. Finally, a human-computer interface allows the network analyst to monitor the sensor trustworthiness or any other data being communicated across the framework.
The figure shows an overview of the FEATS architecture. Classifiers at each node (e.g., sensor, hub) monitor incoming data for anomalous events. In this example, the topology is hierarchical, so each classification tier processes data output from the tier beneath it. Classifiers at higher levels can detect patterns of behavior across multiple sensors or groups of sensors.
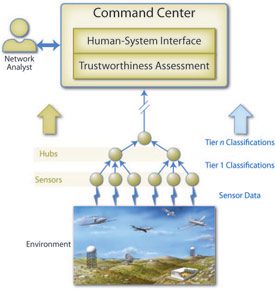
The Benefit
With this framework, a sensor network analyst can monitor the trustworthiness of sensors in the sensor network in real time and proactively take action before questionable data is integrated into mission planning and decision making. With the FEATS framework, the network analyst can quickly design and deploy an anomaly detection application that is customized to the unique characteristics of the deployed sensor network.
Contact us to learn more about FEATS and our other capabilities.
This work was sponsored by the Air Force Research Laboratory under Contract # FA8650-09-C-1612.
UNCLASSIFIED: Distribution Statement A. Approved for public release.