Explainable AI
Scientists and engineers at Charles River Analytics are generating new knowledge at the frontiers of this rapidly growing area, creating a future where humans and AIs collaborate—to drive cars, respond to disasters, and make medical diagnoses.
Why XAI?
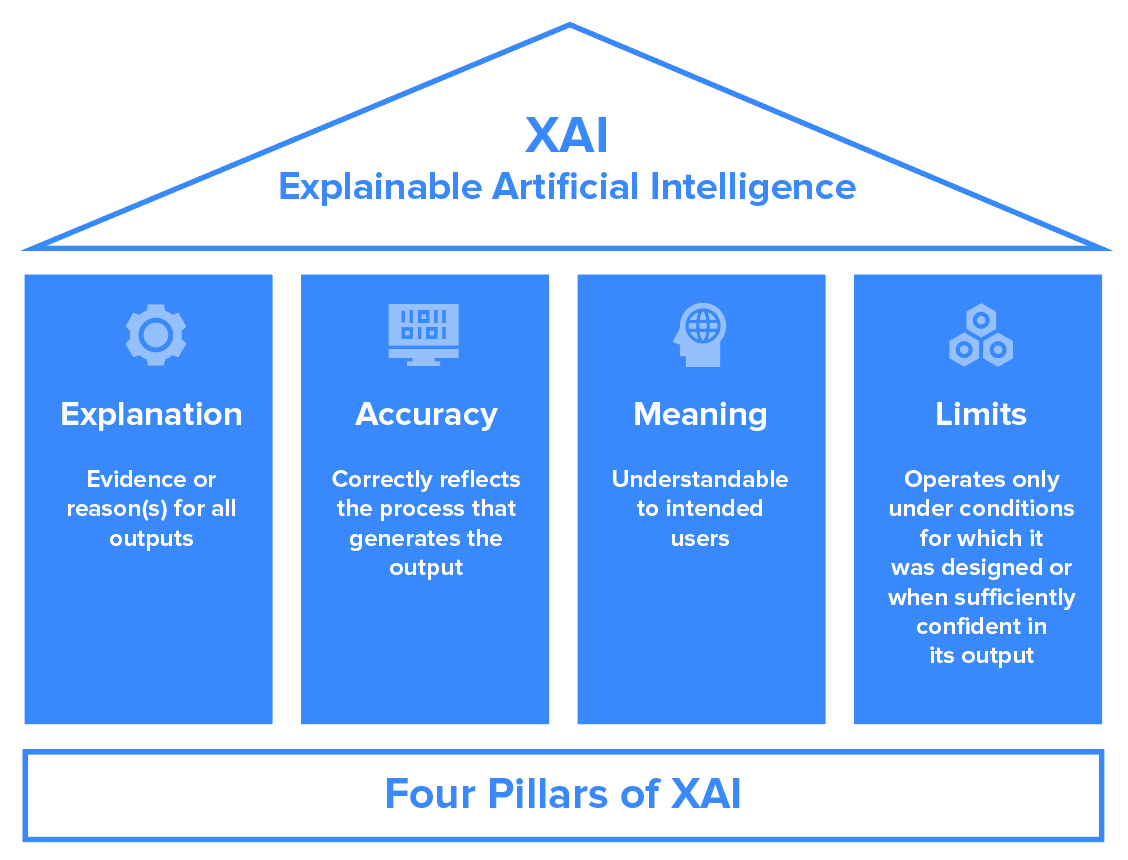
Adapted from Four Principles of XAI, a draft report from the National Institutes of Standards and Technology
Our Approach
CAMEL Project: Using a Real-Time Strategy Game as an Environment for XAI
Our XAI does more than provide correlation-based explanations of AI system outputs: it offers deep insight and causal understanding of the decision-making process. The value of this approach is backed by user studies demonstrating increased trust in the AI system and enhanced human-AI collaboration. Combining our cutting-edge research on XAI with our decades of experience applying AI to real problems for real users, we develop complete systems that work with the entire AI ecosystem—hardware, software, algorithms, individuals, teams, and the environmental context. We can also help you add XAI functionality to your system, supporting your compliance with laws and regulations requiring that decisions from automated systems explain the logic behind those decisions.
Making Machine Learning Trustworthy
Data-driven machine learning is the flavor of the day in artificial intelligence, but the “black box” nature of these systems makes them hard to trust. Charles River Analytics President Karen Harper explains how the new field of explainable AI can help us understand these systems and support informed human decision-making.
Featured Projects
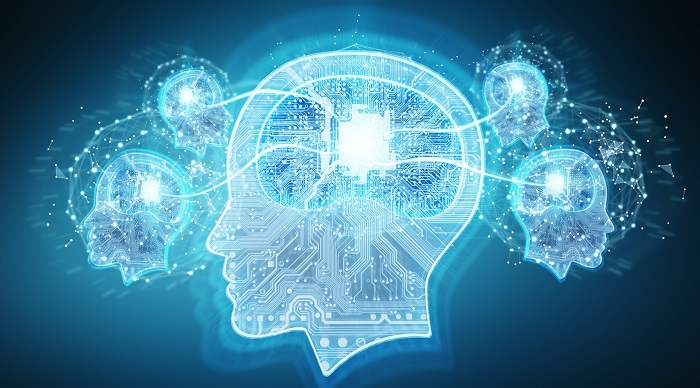
CAMEL
Advancing the emerging field of explainable AI (Part of the DARPA XAI Program)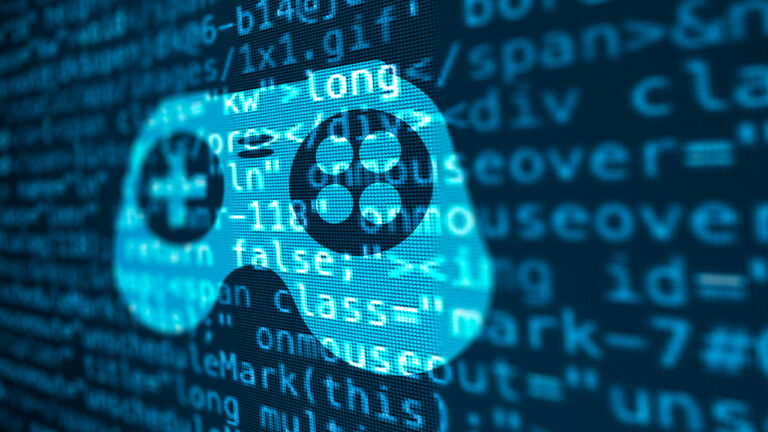
DISCERN
Improve Understanding of Machine Learning (Sponsored by the Office of Naval Research)
ALPACA
A tool that strengthens trust in machine learning (Part of the DARPA CAML Program)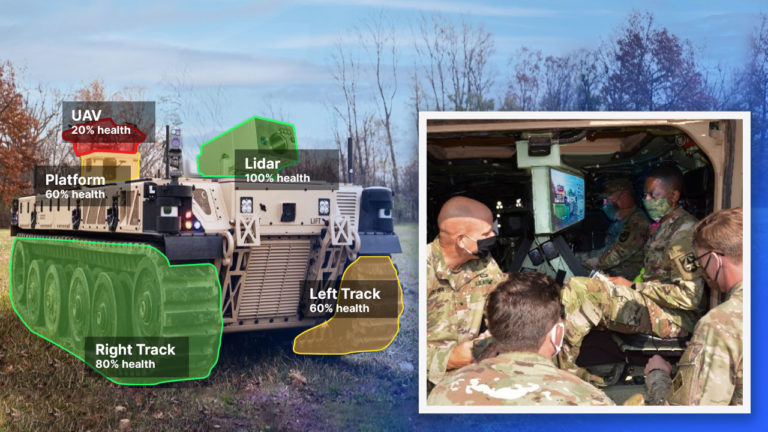
RAPS
An AI-based maintenance system that keeps robotic combat vehicles mission ready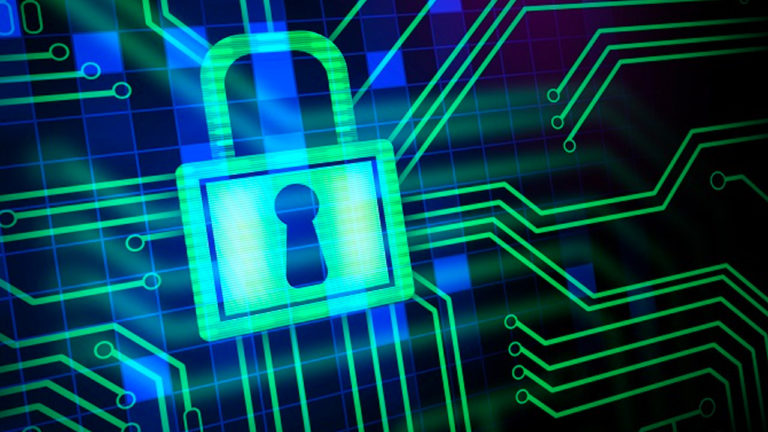
SPNN
Securely train deep neural networks using privacy-prevention encryption.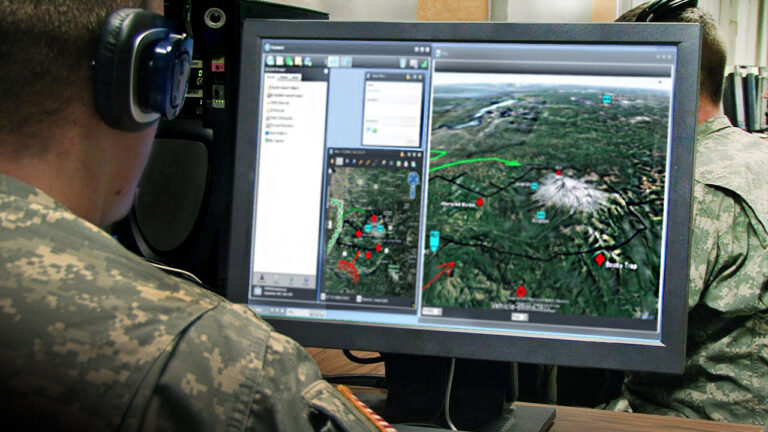
EXTRA
Tools that make DRL agents understandable and trustableA Leading Laboratory
Charles River’s scientists and engineers have been conducting leading-edge research since our founding nearly 40 years ago. Our open, collegial lab collaborates with dozens of universities and research labs across the U.S. We are currently engaged in more than 200 R&D projects, focusing on some of the biggest challenges in AI. Find out more from these selected academic publications and presentations.
Explainable Artificial Intelligence (XAI) for Increasing User Trust in Deep Reinforcement Learning Driven Autonomous Systems
Brittle AI, Causal Confusion, and Bad Mental Models: Challenges and Successes in the XAI Program
From the Press
Our People

Michael Harradon
Senior Scientist
Michael Harradon,
Senior Scientist
Mr. Harradon holds a M.Eng. in Computer Science and a B.S. in Physics and Computer Science, both from MIT, where he also won first place in the 2016 100K Pitch Competition. His primary research interests are machine learning and reinforcement learning (RL), and he is an expert in probabilistic AI, including probabilistic programming. He’s applied novel machine learning approaches to a range of domains, including financial data analysis, radar signals processing, and computer vision. Other areas of expertise include signals analysis, game theory, optimization methods, and genetic algorithms.
Harradon is the inventor of Neural Program Policies (NPPs) and a pioneer in the NPP approach to explainable-by-construction RL agents. As principal investigator for several projects funded under DARPA’s OFFSET program, he is applying NPPs to automatically learn drone swarm behaviors in urban settings. For PERCIVAL-MERLIN, he used NPPs to develop RL-trained agents that predict and track surface vessels.
Harradon has led numerous other projects at Charles River Analytics. As the principal investigator on the STAPLES project (under the DARPA LogX program), he is leading the development of new probabilistic models and inference algorithms for logistics forecasting.
Harradon is a key figure in Charles River’s leadership in the emerging field of explainable AI; he works on explainable modeling and deep RL for the CAMEL effort and he developed novel approaches to identifying human intelligible concepts for the ALPACA project. He is the co-author (with Jeff Druce and Brian Ruttenberg) of “Causal Learning and Explanation of Deep Neural Networks Via Autoencoded Activations” and co-author (with Jeff Druce and James Tittle) of “Explainable Artificial Intelligence (XAI) for Increasing User Trust in Deep Reinforcement Learning Driven Autonomous Systems.”
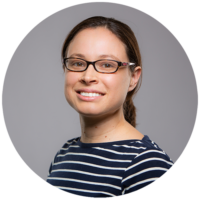
Stephanie Kane
Principal Scientist and Division Director
Stephanie Kane
Principal Scientist
Stephanie Kane is Principal Scientist and Director in the UX Innovations division at Charles River Analytics, an R&D company. Stephanie is a leading researcher of cutting edge user interface and interaction design approaches across a broad range of commercial, government, and educational applications. For over 15 years, she has managed projects over a wide range of technical disciplines for customers such as the Air Force, Navy, Army, and DARPA. Stephanie’s research is focused on the effective design, development, and evaluation of novel user interfaces. She has extensive experience designing and evaluating screen, mobile, tablet, touch, gesture, auditory, voice, and augmented-reality-based interaction methods.

James Niehaus
Principal Scientist and Division Director
James Niehaus
Principal Scientist and Director
Dr. Niehaus specializes in the computational modeling of narrative. As the principal investigator for multiple efforts to develop new training technologies, Niehaus has developed game-based training and virtual coaches for a wide range of audiences; investigated the neural, cognitive, and behavioral relationship between implicit learning and intuition; and worked on social robots for Alzheimer’s patients.
With more than 15 years’ experience applying AI to training systems and instructional design, Niehaus is a highly valued mentor and collaborator for other Charles River scientists and engineers, contributing to a variety of virtual and adaptive systems for medical training. He’s also worked on narrative generation projects, cultural interaction with virtual agents, and cognitive models of discourse comprehension.
Niehaus has a B.S. in computer science from the College of Charleston and a Ph.D. in computer science from North Carolina State University. He is a member of the Society for Simulation in Healthcare and of the National Training and Simulation Association. His extensive publications list includes “Designing Serious Games to Train Medical Team Skills” (with Ashley McDermott and Peter Weyhrauch), “Applications of Virtual Environments in Human Factors Research and Practice” (co-author), and “Development of Virtual Simulations for Medical Team Training: An Evaluation of Key Features” (with Ashley McDermott, Peter Weyhrauch, et al.)