PUBLICATIONS
PUBLICATIONS
A Multi-Agent Trust Framework for Fusing Subjective Opinions with Imperfect Understanding in Space Domain Awareness Using the Scruff AI Framework
Matthew Wilkins1, Sanja Cvijic2, Weston Faber1 Advanced Maui Optical and Space Surveillance Technologies Conference (AMOS), Maui, HI (September 2024) It is widely acknowledged that the space domain is contested and congested. [1] Owing to high dollar outcomes and negative impact
Divergence in situation awareness and workload
Mica R. Endsley1, Jordan Dixon2, Tristan Endsley2, David Jamrog2, Laura Smith-Velazquez3 and Avi Pfeffer3 Ergonomics, (13 Nov 2024) DOI: 10.1080/00140139.2024.2427859. ISSN: (Print) (Online) Journal homepage: www.tandfonline.com/journals/terg20 Situation awareness (SA) and workload have both received considerable attention over the past several decades.
Democratizing AI for Condition-Based Maintenance using Probabilistic Programming
Kenneth Lu1, Sanja Cvijic1, David Dewhurst1, Joe Gorman1, Rob Hyland1, James Templin2 Proceedings of The 69th Annual Reliability & Maintainability Symposium (RAMS®) (January 2023) Advances in AI/ML have demonstrated enormous potential in improving and optimizing condition-based maintenance processes; however, AI/ML
Need for AI in Transformer Diagnostics and Prognostics
Sanja Cvijic1, Nidhi Gupta1, Scott Lux2 Proceedings of The 69th Annual Reliability & Maintainability Symposium (RAMS®) (January 2023) Power equipment, such as electrical transformers, undergo performance degradation over time that can prevent them from functioning properly in critical situations or
Probabilistic Programming: Past, Present, and Future
Pfeffer, A. Invited keynote at the 22nd International Conference on Information Fusion (Fusion 2019), Ottawa, Canada (July 2019) Overview: What is Probabilistic Programming? Probabilistic Programming in Action Probabilistic Programming Inference Algorithms Probabilistic Programming for Long-Lived AI Systems Download Slides For
Structured Factored Inference for Probabilistic Programming
Pfeffer, A., Ruttenberg, B., Kretschmer, W., and O’Connor, A. Presented at the 21st International Conference on Artificial Intelligence and Statistics (AISTATS 2018), Lanzarote, Canary Islands (April 2018) Probabilistic reasoning on complex real-world models is computationally challenging. Inference algorithms have been
Scruff: A Deep Probabilistic Cognitive Architecture
Pfeffer, A. Invited talk at the Association for the Advancement of Artificial Intelligence’s Thirty-Second AAAI Conference on Artificial Intelligence (AAAI-18), New Orleans, LA (February 2018). Probabilistic programming is able to build rich models of systems that combine prior knowledge with
Using Reinforcement Learning for Probabilistic Program Inference
Pfeffer, A. Extended abstract for the Probabilistic Program Semantics Workshop associated with the Principles of Programming Languages (POPL) conference, Los Angeles, CA (January 2018). Inference in probabilistic programming often involves choosing between different methods. For example, one could use different
Probabilistic Model-Based Programming Techniques for Prediction, Analysis, and Control (PROMPT)
Harrison, S., Takata, G., Wu, C., and Pfeffer, A. Presented at the 15th Annual Conference on Systems Engineering Research Disciplinary Convergence: Implications for Systems Engineering Research, Redondo Beach, CA (March 2017) Model-based systems engineering (MBSE) frameworks such as SysML provide declarative descriptions
Practical Probabilistic Programming
Pfeffer, A. Practical Probabilistic Programming, Manning Publications, Cherry Hill, NJ (2016) Practical Probabilistic Programming introduces the working programmer to probabilistic programming. In it, you’ll learn how to use the PP paradigm to model application domains and then express those probabilistic models in
SEARCH/FILTER
SEARCH/FILTER
A Multi-Agent Trust Framework for Fusing Subjective Opinions with Imperfect Understanding in Space Domain Awareness Using the Scruff AI Framework
Matthew Wilkins1, Sanja Cvijic2, Weston Faber1 Advanced Maui Optical and Space Surveillance Technologies Conference (AMOS), Maui, HI (September 2024) It is widely acknowledged that the space domain is contested and congested. [1] Owing to high dollar outcomes and negative impact
Divergence in situation awareness and workload
Mica R. Endsley1, Jordan Dixon2, Tristan Endsley2, David Jamrog2, Laura Smith-Velazquez3 and Avi Pfeffer3 Ergonomics, (13 Nov 2024) DOI: 10.1080/00140139.2024.2427859. ISSN: (Print) (Online) Journal homepage: www.tandfonline.com/journals/terg20 Situation awareness (SA) and workload have both received considerable attention over the past several decades.
Democratizing AI for Condition-Based Maintenance using Probabilistic Programming
Kenneth Lu1, Sanja Cvijic1, David Dewhurst1, Joe Gorman1, Rob Hyland1, James Templin2 Proceedings of The 69th Annual Reliability & Maintainability Symposium (RAMS®) (January 2023) Advances in AI/ML have demonstrated enormous potential in improving and optimizing condition-based maintenance processes; however, AI/ML
Need for AI in Transformer Diagnostics and Prognostics
Sanja Cvijic1, Nidhi Gupta1, Scott Lux2 Proceedings of The 69th Annual Reliability & Maintainability Symposium (RAMS®) (January 2023) Power equipment, such as electrical transformers, undergo performance degradation over time that can prevent them from functioning properly in critical situations or
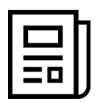
Probabilistic Programming: Past, Present, and Future
Pfeffer, A. Invited keynote at the 22nd International Conference on Information Fusion (Fusion 2019), Ottawa, Canada (July 2019) Overview: What is Probabilistic Programming? Probabilistic Programming in Action Probabilistic Programming Inference Algorithms Probabilistic Programming for Long-Lived AI Systems Download Slides For
Structured Factored Inference for Probabilistic Programming
Pfeffer, A., Ruttenberg, B., Kretschmer, W., and O’Connor, A. Presented at the 21st International Conference on Artificial Intelligence and Statistics (AISTATS 2018), Lanzarote, Canary Islands (April 2018) Probabilistic reasoning on complex real-world models is computationally challenging. Inference algorithms have been
Scruff: A Deep Probabilistic Cognitive Architecture
Pfeffer, A. Invited talk at the Association for the Advancement of Artificial Intelligence’s Thirty-Second AAAI Conference on Artificial Intelligence (AAAI-18), New Orleans, LA (February 2018). Probabilistic programming is able to build rich models of systems that combine prior knowledge with
Using Reinforcement Learning for Probabilistic Program Inference
Pfeffer, A. Extended abstract for the Probabilistic Program Semantics Workshop associated with the Principles of Programming Languages (POPL) conference, Los Angeles, CA (January 2018). Inference in probabilistic programming often involves choosing between different methods. For example, one could use different
Probabilistic Model-Based Programming Techniques for Prediction, Analysis, and Control (PROMPT)
Harrison, S., Takata, G., Wu, C., and Pfeffer, A. Presented at the 15th Annual Conference on Systems Engineering Research Disciplinary Convergence: Implications for Systems Engineering Research, Redondo Beach, CA (March 2017) Model-based systems engineering (MBSE) frameworks such as SysML provide declarative descriptions
Practical Probabilistic Programming
Pfeffer, A. Practical Probabilistic Programming, Manning Publications, Cherry Hill, NJ (2016) Practical Probabilistic Programming introduces the working programmer to probabilistic programming. In it, you’ll learn how to use the PP paradigm to model application domains and then express those probabilistic models in
Partners in Research
Frequently colaborating with leading organizations
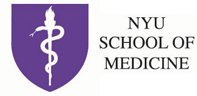
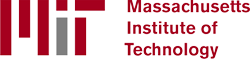
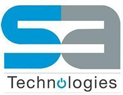
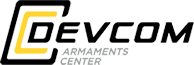

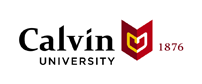
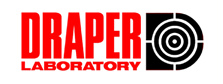
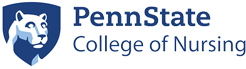

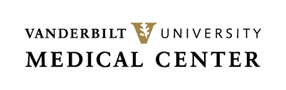
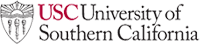
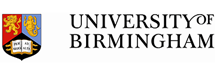
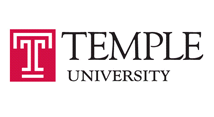
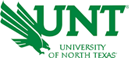
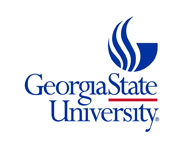