A Multi-Agent Trust Framework for Fusing Subjective Opinions with Imperfect Understanding in Space Domain Awareness Using the Scruff AI Framework
Matthew Wilkins1, Sanja Cvijic2, Weston Faber1 Advanced Maui Optical and Space Surveillance Technologies Conference (AMOS), Maui, HI (September 2024) It is widely acknowledged that the space domain is contested and congested. [1] Owing to high dollar outcomes and negative impact to critical missions, agents in the space domain rarely accept data from untrusted sources (i.e. […]
Divergence in situation awareness and workload
Mica R. Endsley1, Jordan Dixon2, Tristan Endsley2, David Jamrog2, Laura Smith-Velazquez3 and Avi Pfeffer3 Ergonomics, (13 Nov 2024) DOI: 10.1080/00140139.2024.2427859. ISSN: (Print) (Online) Journal homepage: www.tandfonline.com/journals/terg20 Situation awareness (SA) and workload have both received considerable attention over the past several decades. Little research has investigated the relationship between these two constructs however. The present study examines […]
Democratizing AI for Condition-Based Maintenance using Probabilistic Programming
Kenneth Lu1, Sanja Cvijic1, David Dewhurst1, Joe Gorman1, Rob Hyland1, James Templin2 Proceedings of The 69th Annual Reliability & Maintainability Symposium (RAMS®) (January 2023) Advances in AI/ML have demonstrated enormous potential in improving and optimizing condition-based maintenance processes; however, AI/ML solutions themselves inevitably become a maintenance liability, wherein the end users must repeatedly work with […]
Need for AI in Transformer Diagnostics and Prognostics
Sanja Cvijic1, Nidhi Gupta1, Scott Lux2 Proceedings of The 69th Annual Reliability & Maintainability Symposium (RAMS®) (January 2023) Power equipment, such as electrical transformers, undergo performance degradation over time that can prevent them from functioning properly in critical situations or even cause catastrophic failures. Improved diagnostic and prognostic approaches that more accurately determine the reliability […]
Probabilistic Programming: Past, Present, and Future
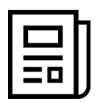
Pfeffer, A. Invited keynote at the 22nd International Conference on Information Fusion (Fusion 2019), Ottawa, Canada (July 2019) Overview: What is Probabilistic Programming? Probabilistic Programming in Action Probabilistic Programming Inference Algorithms Probabilistic Programming for Long-Lived AI Systems Download Slides For More Information To learn more, contact Avi Pfeffer. (Please include your name, address, organization, and […]
Structured Factored Inference for Probabilistic Programming
Pfeffer, A., Ruttenberg, B., Kretschmer, W., and O’Connor, A. Presented at the 21st International Conference on Artificial Intelligence and Statistics (AISTATS 2018), Lanzarote, Canary Islands (April 2018) Probabilistic reasoning on complex real-world models is computationally challenging. Inference algorithms have been developed that work well on specific models or on parts of general models, but they […]
Scruff: A Deep Probabilistic Cognitive Architecture
Pfeffer, A. Invited talk at the Association for the Advancement of Artificial Intelligence’s Thirty-Second AAAI Conference on Artificial Intelligence (AAAI-18), New Orleans, LA (February 2018). Probabilistic programming is able to build rich models of systems that combine prior knowledge with the ability to learn from data. One of the reasons for the success of deep […]
Using Reinforcement Learning for Probabilistic Program Inference
Pfeffer, A. Extended abstract for the Probabilistic Program Semantics Workshop associated with the Principles of Programming Languages (POPL) conference, Los Angeles, CA (January 2018). Inference in probabilistic programming often involves choosing between different methods. For example, one could use different algorithms to compute a conditional probability, or one could sample variables in different orders. Researchers […]
Probabilistic Model-Based Programming Techniques for Prediction, Analysis, and Control (PROMPT)
Harrison, S., Takata, G., Wu, C., and Pfeffer, A. Presented at the 15th Annual Conference on Systems Engineering Research Disciplinary Convergence: Implications for Systems Engineering Research, Redondo Beach, CA (March 2017) Model-based systems engineering (MBSE) frameworks such as SysML provide declarative descriptions of the structure, processes, functions, and context of a system. However, these frameworks do not […]
Practical Probabilistic Programming
Pfeffer, A. Practical Probabilistic Programming, Manning Publications, Cherry Hill, NJ (2016) Practical Probabilistic Programming introduces the working programmer to probabilistic programming. In it, you’ll learn how to use the PP paradigm to model application domains and then express those probabilistic models in code. Although PP can seem abstract, in this book you’ll immediately work on practical examples, […]