Brittle AI, Causal Confusion, and Bad Mental Models: Challenges and Successes in the XAI Program
Druce, J.1, Niehaus, J.2, Moody, V.1, Jensen, D.2, Littman, M.3 arXiv:2106.05506v1 (June 2021) The advances in artificial intelligence enabled by deep learning architectures are undeniable. In several cases, deep neural network driven models have surpassed human level performance in benchmark autonomy tasks. The underlying policies for these agents, however, are not easily interpretable. In fact, […]
Evaluation of an AI System Explanation Interface
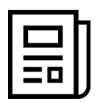
Tittle, J.1, Niehaus, J.1, Druce, J.1, Harradon, M.1, Roth, E.2, and Voshell, M.1 14th International Naturalistic Decision Making Conference, San Francisco, CA (June 2019) Designing effective explainable artificial intelligence (XAI) systems represents a fundamental challenge for trusted and cooperative human machine collaboration. In this paper we describe the development and evaluation of an explanation interface that […]
Causal Learning and Explanation of Deep Neural Networks via Autoencoded Activations
Harradon, M., Druce, J., and Ruttenberg, B. arXiv:1802.00541v1 (February 2018) Deep neural networks are complex and opaque. As they enter application in a variety of important and safety critical domains, users seek methods to explain their output predictions. We develop an approach to explaining deep neural networks by constructing causal models on salient concepts contained […]