DATEM
Monitor health and status of critical systems with machine-learning technologies
Monitor health and status of critical systems with machine-learning technologies
Distributed Analysis Tool for Enterprise Monitoring (DATEM)
DATEM is a real-time alerting system that provides tripwires to detect, classify, and localize system faults. DATEM identifies inappropriate operator behaviors (e.g., failure to respond to critical system alerts), recognizes emergent conditions of interest (e.g., hardware or software faults, resource limits), and recommends appropriate responses to improve mission’s success. DATEM makes sense of health and status data and alerts Sailors of abnormal system behavior.
“It’s extremely humbling—and very exciting—to know DATEM will be used daily by the Navy,” explained Joe Gorman, Charles River’s Principal Investigator on the DATEM effort. “Our system also provides an opportunity for us to apply our latest and greatest machine learning research for fault detection and classification with probabilistic modeling for fault localization. The combination of fault detection and localization technologies improves efficiency and makes DATEM valuable to the Navy.”
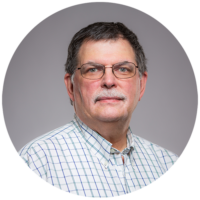
Joseph Gorman,
Principal Software Engineer and Principal Investigator on DATEM effort
The Navy has developed numerous net-centric information systems that support fleet operations. Adverse operational conditions, operator behaviors, and routine wear all affect the availability and performance of these systems. For example, high temperatures may lead to a part failure that degrades performance. Worse, some failures are very difficult to detect and classify. These conditions challenge Sailors’ ability to respond rapidly and effectively to failures and maintain mission readiness.
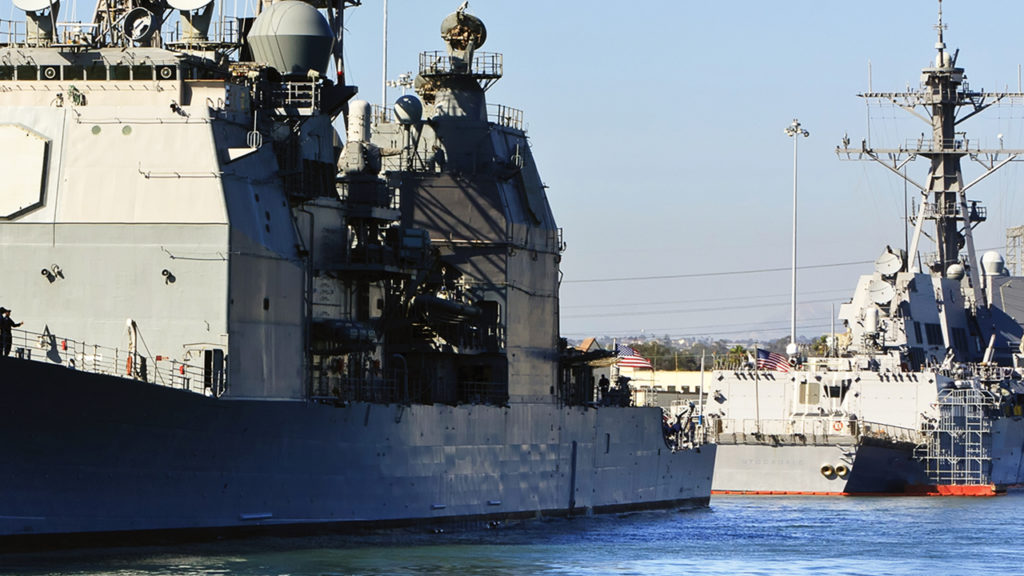
In our prior research, we delivered an automated fault classifier that significantly improved the efficiency and accuracy of previous semi-automatic fault analysis approaches. Then, we used models to automatically localize sources of failure to field replaceable units. These models drastically reduced the time it takes for Sailors to discover and resolve faults.
DATEM is another successful application of Figaro™, our probabilistic programming language. While DATEM is currently available to provide real-time fault detection and localization to ship support specialists, Figaro will allow us to significantly improve the effectiveness of its fault localization feature.
Contact us to learn more about DATEM and our other AI capabilities.
This material is based upon work supported by the Space and Naval Warfare Systems Command under Contract No. N68335-18-C-0358. Any opinions, findings and conclusions or recommendations expressed in this material are those of the author(s) and do not necessarily reflect the views of the Space and Naval Warfare Systems Command.