COLTRANE
A system that uses machine learning and symbolic reasoning to
handle novelty in open-world domains
A system that uses machine learning and symbolic reasoning to handle novelty in open-world domains
Compositionally Organized Learning to Reason about Novel Experiences (COLTRANE)
To create more robust AI systems, we are developing the Compositionally Organized Learning to Reason about Novel Experiences (COLTRANE). COLTRANE combines machine learning and symbolic reasoning so AI systems can adapt to real-world changes whenever and wherever they occur.
Under the Defense Advanced Research Projects Agency’s (DARPA’s) Science of Artificial Intelligence and Learning for Open-world Novelty (SAIL-ON) program, COLTRANE helps AI systems navigate novel situations, such as operating in unfamiliar terrain or reacting to an opponent’s unexpected actions. Researchers from the MIT Department of Brain and Cognitive Science are collaborating with us under the program to apply basic research to solve DARPA evaluation problems.
“The key insight for COLTRANE is that knowledge is both compositional and hierarchical. By maintaining uncertainty about this knowledge and representing it probabilistically through probabilistic programming-based inference, probabilistic program synthesis, and hierarchical planning, we can identify, characterize, and adapt to the novelty the system encounter.”
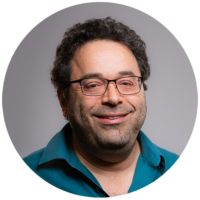
Dr. Avi Pfeffer,
Chief Scientist at Charles River
“Current AI systems are brittle to change; this limits their applicability, leads to expensive engineering efforts, and results in unpredictable failures,” said Dr. Bryan Loyall, Director of Technology Innovation at Charles River. “COLTRANE merges symbolic and sub-symbolic reasoning so AI can operate effectively in open worlds.”
Just like a jazz musician who improvises novel melodies by combining elements of musical structure, COLTRANE synthesizes new representations by combining basic elements into new concepts. COLTRANE then reasons about change using the new representations to adapt in real time. Unlike traditional AI machine learning systems that require large amounts of training data for every situation, COLTRANE uses its knowledge structures to learn about new situations from a very small set of training instances, then applies the structures again to adapt on the fly.
COLTRANE’s automatic adaptation makes AI systems safer and more effective; engineers no longer have to rework these systems every time there’s a change in the environment.
Related Contract and Publication
Coltrane: A domain-independent system for characterizing and planning in novel situations
Contact us to learn more about COLTRANE and our other AI and probabilistic modeling/programming capabilities.
This material is based upon work supported by the Defense Advanced Research Projects Agency (DARPA) under Contract No. HR001120C0042. Any opinions, findings and conclusions or recommendations expressed in this material are those of the author(s) and do not necessarily reflect the views of the DARPA.