AUTHOR
Tool to determine authorship of anonymous documents
Tool to determine authorship of anonymous documents
Attribution of, and Undermining the Attribution of, Text while providing Human-Oriented Rationales (AUTHOR)
The Human Interpretable Attribution of Text Using Underlying Structure (HIATUS) program sponsored by IARPA uses natural language processing techniques and machine learning to create stylistic fingerprints that capture the writing style of specific authors.
On the flip side is authorship privacy, mechanisms that can anonymize identities of authors, especially when their lives are in danger.
While authorship attribution has usually looked for words and their frequencies as identifying fingerprints, AUTHOR evaluates grammar as well, such as the use of passive voice or nominalization. AUTHOR’s set of attribution parameters also includes discourse features—how authors structure their arguments.
“One of the big challenges for the program and for authorship attribution in general is that the document you’re looking at may not be in the same genre or on the same topic as the sample documents you have for a particular author.
The same applies to languages: We might have example articles for an author in English but need to match the style even if the document at hand is in French. Authorship privacy also has its challenges: Users must obfuscate the style without changing the meaning, which can be difficult to execute.”
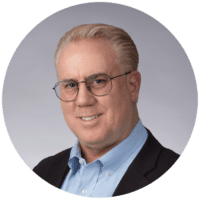
Dr. Terry Patten,
Principal Scientist and Principal Investigator on AUTHOR
Forensic Linguistics
The growth of natural language processing (NLP) and one of its underlying techniques, machine learning, is motivating researchers to harness these new technologies in solving the classic problem of authorship attribution. The challenge is that while machine learning is very effective at authorship attribution, deep learning systems that use neural networks can’t explain why they arrived at the answers they did.
Evidence in criminal trials can’t afford to hinge on such black-box systems. It’s why the core condition of AUTHOR is that it be “human-interpretable.” Deep learning’s black box problem has been well documented, so figuring out how to arrive at human-interpretable explanations will contribute to the larger AI field as well.
The project is initially focusing on feature discovery: Beyond words, what features can we discover to increase the accuracy of authorship attribution? The project has a range of promising applications – identifying counterintelligence risks, combating misinformation online, fighting human trafficking, and even figuring out authorship of ancient religious texts.
Patten is excited about the promise of AUTHOR as it is poised to make fundamental contributions to the field of NLP. “It’s really forcing us to address an issue that’s been central to natural language processing,” Patten says. “In NLP and AI in general, we need to find a way to build hybrid systems that can incorporate both deep learning and human-interpretable representations. The field needs to find ways to make neural networks and linguistic representations work together.”
The team includes some of the world’s foremost researchers in authorship analysis, computational linguistics, and machine learning from Illinois Institute of Technology, Aston Institute for Forensic Linguistics, Rensselaer Polytechnic Institute, and Howard Brain Sciences Foundation.
Contact us to learn more about AUTHOR and our other AI capabilities.
This research is supported in part by the Office of the Director of National Intelligence (ODNI), Intelligence Advanced Research Projects Activity (IARPA), via the HIATUS Program contract #2022-2207220001. The views and conclusions contained herein are those of the authors and should not be interpreted as necessarily representing the official policies, either expressed or implied, of ODNI, IARPA, or the U.S. Government. The U.S. Government is authorized to reproduce and distribute reprints for governmental purposes notwithstanding any copyright annotation therein.