You’re a small-unit leader defending a strategic fortified position from an approaching enemy. You don’t have the manpower to do it on your own, but an unmanned ground vehicle (UGV) could provide the logistics and reconnaissance support you need. The UGV is controlled by an artificial intelligence (AI) that performs effectively on average, but it can make inexplicable errors that lead to catastrophic results. Without knowing when and whether to trust this potential teammate, you can’t take the risk of relying on it.
CAMEL (Causal Models to Explain Learning) aims to change this scenario, using cutting-edge advances in the emerging field of explainable AI (XAI).
In today’s AI systems based on deep reinforcement learning (RL), the RL agent’s decision-making process is a “black box.” As a result, some users try to explain system outcomes with incorrect “folk theories,” which can lead to dangerous outcomes. CAMEL addresses this problem by providing accurate, understandable explanations of agent decisions.
The Charles River project team demonstrated that the CAMEL approach led to enhanced user trust and system acceptance when classifying images of pedestrians. Then, they took on a more exciting challenge—human-machine teaming for the real-time computer strategy game StarCraft II.
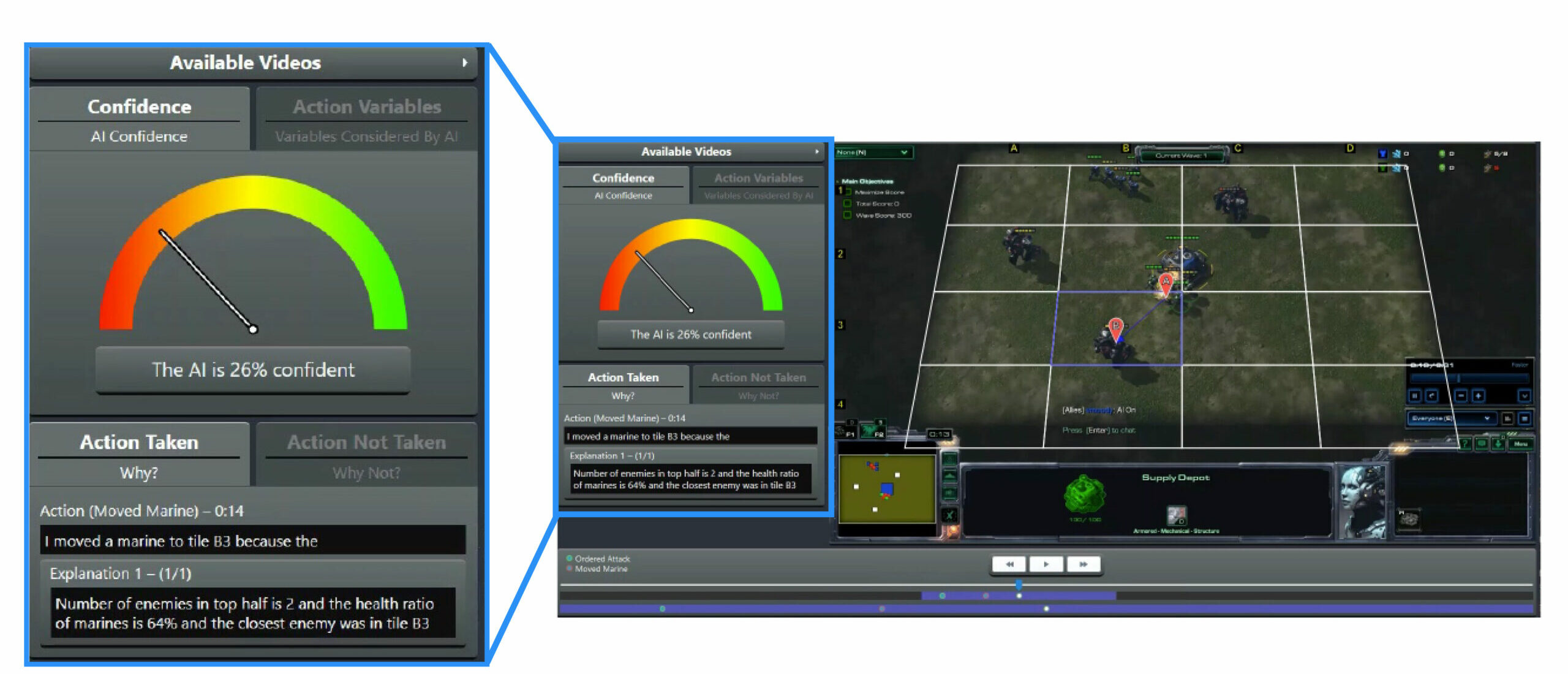
CAMEL’s explanations are based on causal models, which describe how one part of a system influences other parts of the system. These models help determine the real driving forces of system behavior. Using this information, CAMEL creates counterfactuals—explanations of the agent’s behavior when it’s not what the user expected. For example, a user asks, “Why did the agent attack?” and CAMEL’s Explanation User Interface answers, “If the health of enemy-294 had been 50% higher, the agent would have retreated.”
Using CAMEL explanations, StarCraft II players created more accurate mental models of the RL agent, and used this improved understanding to achieve higher game scores.
So how would CAMEL change the opening scenario and help the small-unit leader? “CAMEL’s explanations are not a ‘one-off’ exposure to the system,” said Dr. Jeff Druce, Senior Scientist at Charles River Analytics and Principal Investigator of the CAMEL effort. “Commanders, decision-makers, and teammates can use CAMEL when training with complex AIs.” Through this training, users will learn when AIs can be trusted. Knowing the UGV’s capabilities, the small-team leader can deploy it effectively for mission support.
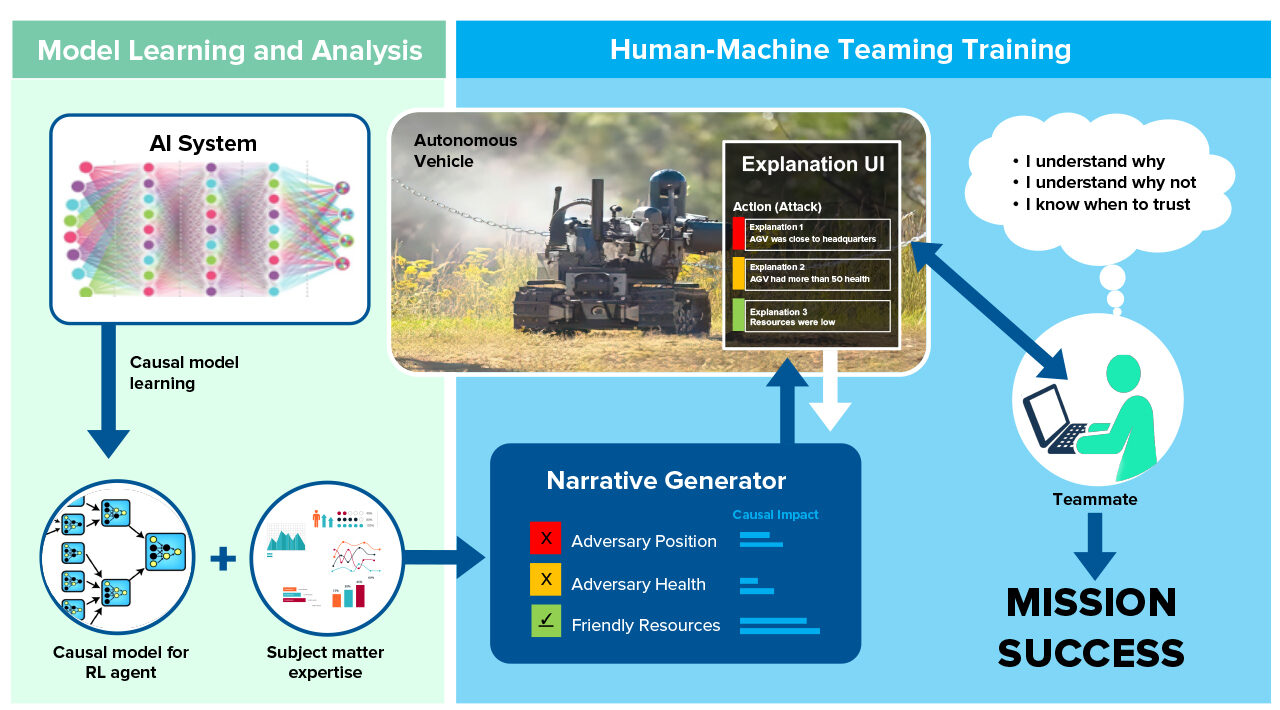
Charles River developed CAMEL as part of the XAI program for the Defense Advanced Research Project Agency (DARPA). Through the XAI program, DARPA is funding multiple efforts to make AI more explainable by combining machine learning (ML) techniques with human-computer interaction design. The four-year contract is valued at close to $8 million. CAMEL will significantly affect the way that machine learning systems are deployed, operated, and used inside and outside the Department of Defense, and is poised to address explainability and human-machine teaming in new and challenging domains, such Intelligence, Surveillance, and Reconnaissance (ISR) and Command and Control (C2).
The development of CAMEL draws on Charles River’s expertise in machine learning, causal modeling, and human factors. The project is a cornerstone of Charles River’s emerging leadership in XAI. Other projects in this domain include ALPACA and DISCERN.
Charles River Analytics conducts cutting-edge AI, robotics, and human-machine interface R&D. Our solutions enrich the diverse markets of defense, intelligence, medical technology, training, transportation, space, and cybersecurity. Our customer focus guides us towards problems that matter, while our passion for science and engineering drives us to innovate.
This material is based upon work supported by the Defense Advanced Research Projects Agency (DARPA) under Contract No. FA8750-17-C-0118. Any opinions, findings and conclusions or recommendations expressed in this material are those of the author(s) and do not necessarily reflect the views of DARPA. Distribution Statement “A” (Approved for Public Release, Distribution Unlimited). The appearance of U.S. Department of Defense (DoD) visual information does not imply or constitute DoD endorsement.