Charles River Analytics received a $1.8 million contract to classify and identify ships and other vessels at sea. The Automatic Text Extraction for Awareness in the Maritime (A-TEAM) system works with existing labeled image datasets and banks of unannotated maritime images to enable automated systems to recognize text on ships at sea.
Knowing the ship’s name and International Maritime Organization (IMO) number will help the Navy identify threat actors more easily and conduct efficient surveillance on water. The ability to go beyond just detecting and classifying to actually identifying the ships is an extension of the Awarion® product that Charles River Analytics has been developing in parallel. “It has been exciting to apply the team’s maritime expertise to a new challenge,” says Ross Eaton, Principal Scientist and Director of Marine Systems at Charles River Analytics and Principal Investigator on A-TEAM.
Translating a vision skill, such as text recognition, that was learned from land-based data to sea-based imagery involves domain adaptation—a kind of machine learning. To save time and resources, the Navy is looking to work with a large set of unannotated imagery, which would classify the project as unsupervised domain adaptation.
Optical character recognition (OCR), the technology used to automatically read lettering and numbers, is a well-established technique, but its use at sea presents unique challenges. First, there is the question of when exactly to aim the cameras at the lettering to obtain effective results. “Ships are huge and the letters on them are small in comparison, so you can tell there’s a ship long before you have a chance to read anything on it,” Eaton says. At long ranges, additional problems such as atmospheric turbulence tend to blur and distort the shape of faraway lettering. Fortunately, another tool designed by Charles River helps correct blur and improve image quality in the preprocessing stage to make OCR more accurate.
A second challenge is that text recognition systems are not trained on structures unique to ships. To the untrained eye, a series of portholes might look like a cluster of the letter “O” strung together. In addition, the curves of a ship’s hull and the ridges on a cargo container can distort the shape of letters printed on them. Understanding what a letter looks like under ideal conditions is not enough for the algorithm to do its job at sea.
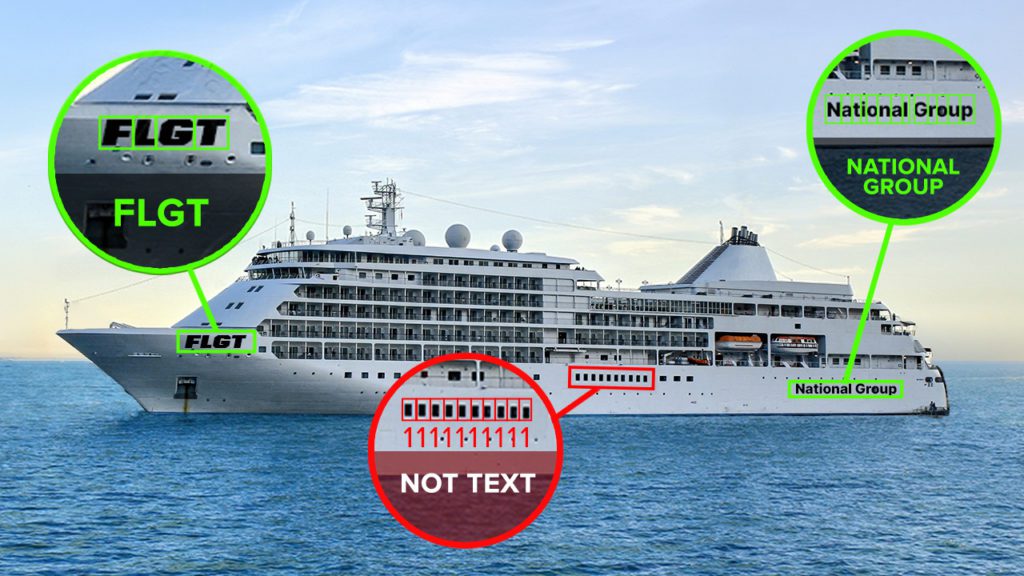
Despite these challenges, text recognition algorithms continue to improve, and with augmentations developed under A-TEAM, they are performing quite well on maritime data, Eaton says. “Charles River’s Awarion maritime perception tool could already detect and classify ships. By integrating this text recognition capability, it can now identify specific ships at sea to track longer-term behavior and build models to determine when a ship is behaving in an unusual manner.”
Eaton expects A-TEAM to eventually incorporate multi-sensor data—from radar and cameras, for example—to build an even more cohesive picture for identification. “This aspect of the tool could be especially valuable for helping crews on vessels to have a more complete picture rather than relying on radar alone,” Eaton says.
A-TEAM demonstrates Charles River’s success in integrating novel technologies into solutions across a wide range of domains. By applying new ideas in domain adaptation and machine learning to its strengths in maritime perception, Charles River is able to build better solutions for their customers. “Our ability to combine our existing intellectual property with leading-edge science to complement our tools and bridge any gaps lets us deliver a product that’s even better than what the customer is expecting,” Eaton says. “Ultimately, it’s of real benefit to the customer.”
Contact us to learn more about A-TEAM and our capabilities in maritime systems and robotics and autonomy.
Effort sponsored by the US Government under Other Transactions number N65236-18-9-0001 between Information Warfare Research Project (IWRP) and the Government. The views and conclusions contained herein are those of the authors and should not be interpreted as necessarily representing the official policies or endorsements, either expressed or implied, of the US Government.